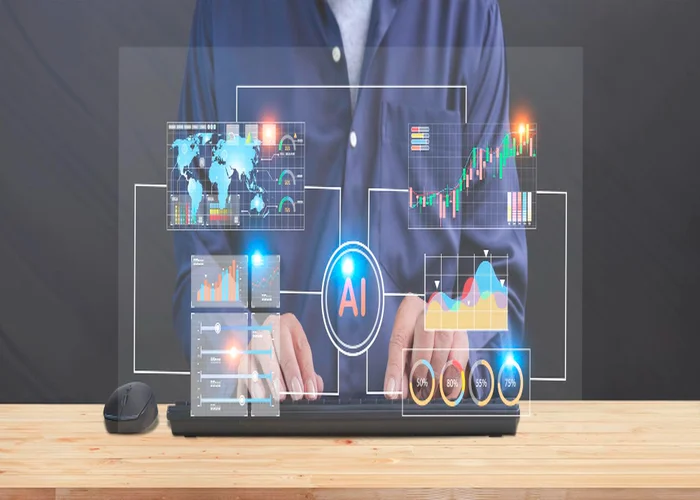
Rise and Importance of Data Science and Artificial Intelligence
The rise of data science and artificial intelligence (AI) has revolutionized the way we handle and interpret data, driving significant advancements across various industries. With the explosion of big data, the integration of AI and data science has become indispensable for extracting valuable insights and making informed decisions. AI data analytics plays a crucial role in this transformation, allowing organizations to analyze vast datasets efficiently and accurately.
Data science tools, enhanced by AI capabilities, enable businesses to uncover hidden patterns and predict future trends, thus facilitating strategic planning and innovation. The combination of data science and machine learning has led to the development of sophisticated models that can learn and adapt over time, improving their performance with each iteration. This synergy between data science and artificial intelligence is fostering the creation of intelligent systems that automate complex tasks, reducing human error and increasing productivity.
Artificial intelligence data science is also driving new developments in various fields, from healthcare and finance to marketing and manufacturing. AI-powered data science tools are helping organizations optimize operations, enhance customer experiences, and create personalized solutions. As artificial intelligence development continues to evolve, the importance of data science and AI in shaping the future of technology and industry becomes ever more apparent, underscoring their role as pivotal drivers of modern innovation.
1. Generative AI: Bridging the Gap to Value
Challenges in Value Delivery
Organizational Adaptation
Benefits and Expectations
2. Industrialization of Data Science
Automation and Efficiency
Technological Infrastructure
MLOps Systems
Role Evolution
As data science becomes more industrialized, the role of data scientists is evolving. Automation and standardized processes enable data scientists to focus on high-value tasks such as developing new algorithms and exploring novel data sources. This shift not only increases the efficiency of data science teams but also democratizes access to data science capabilities, allowing a broader range of stakeholders to participate in data-driven decision-making.
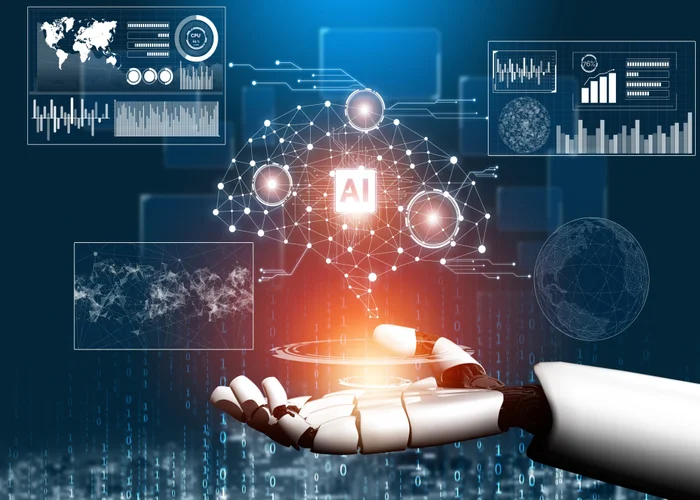
3. Diverging Definitions of Data Products
Defining Data Products
Examples and Applications
Implementation Strategies
Management and Improvement
4. Evolution of Data Scientist Roles
Specialization and Automation
New Roles and Responsibilities
Citizen Data Science
Critical Expertise
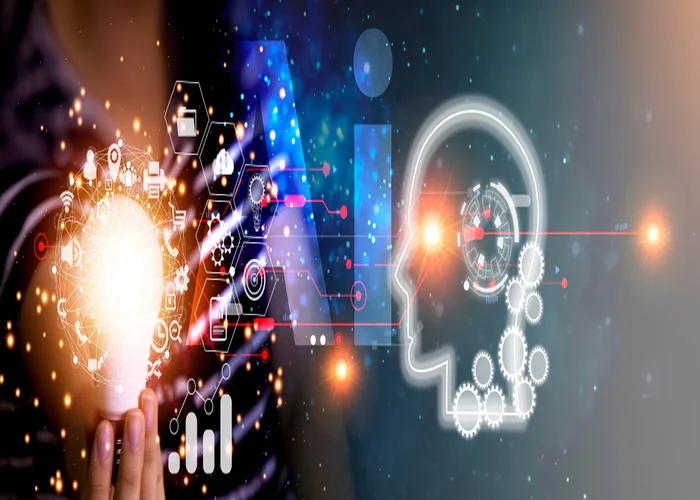
5. Integration of Data and Technology Leadership
Leadership Consolidation
Strategic Alignment
Resource Allocation
Collaboration and Innovation
Collaboration is a key focus of this integrated leadership model. By breaking down silos and fostering cross-functional teamwork, organizations can leverage diverse expertise and perspectives to drive innovation. Senior technology leaders play a crucial role in building a collaborative culture, encouraging open communication, and ensuring that all stakeholders are engaged in the digital transformation journey.
As we progress through 2024, these trends underscore the ongoing evolution and maturation of AI and data science. The promise of generative AI, the shift towards industrialized data science, clarity in defining data products, the evolving role of data scientists, and the integration of leadership functions all point to a dynamic and transformative year ahead. Organizations must stay agile, invest wisely, and adapt to these evolving trends to fully harness the potential of AI and data science.
Shamla Tech: Leading the Way in AI and Data Science Innovation for 2024
At Shamla Tech, our expertise in AI and data science positions us at the forefront of industry advancements, perfectly aligned with the transformative trends shaping 2024. We specialize in harnessing the power of AI and data science to address the evolving needs of businesses, leveraging advanced AI data analytics and cutting-edge data science tools. Our focus on industrializing data science processes, including robust AI data analytics and machine learning operations (MLOps) systems, enables us to deliver scalable and efficient solutions. As leaders in artificial intelligence development, we recognize the transformative potential of generative AI and its role in shaping the future of industries. Our approach emphasizes clarity in defining and managing data products, ensuring that analytics and AI capabilities are optimized for maximum impact.
We are at the forefront of defining the future of AI and data science, empowering businesses to navigate complexities with confidence. Through our expertise in data science tools and machine learning, we enable organizations to unlock actionable insights and drive informed decision-making. By integrating data and technology leadership, we foster collaboration and alignment across organizational functions, driving sustainable growth and competitive advantage.
In 2024 and beyond, Shamla Tech remains dedicated to advancing the frontier of AI and data science. With a focus on innovation, strategic partnership, and client-centric solutions, we continue to empower businesses to thrive in an increasingly digital and data-driven world. Partner with us to harness the full potential of AI and data science, and embark on a journey of continuous transformation and success.