Role of AI in Healthcare
Drug Discovery
Generative AI in Healthcare holds significant promise for drug discovery in healthcare. According to Forbes, generative AI can streamline the drug discovery process by predicting interactions between drugs and specific proteins or targets. Utilizing machine learning algorithms, generative AI analyzes vast datasets and generates novel molecules that could serve as potential drugs much faster than traditional methods. This acceleration can reduce both the time and cost of drug discovery, paving the way for more effective treatments for various diseases.
Medical Imaging
Generative AI in healthcare has the potential to greatly enhance medical imaging by producing synthetic images that are comparable to real images but with reduced noise and quicker scan times. For instance, researchers can employ generative AI to create synthetic MRI images, aiding doctors in making more accurate diagnoses and developing better treatment plans.
Traditional MRI scans often contain a significant amount of noise, making it challenging to detect subtle differences or abnormalities. Artificial Intelligence in healthcare can be used to generate synthetic MRI images that reduce this noise, resulting in clearer and more precise images. Furthermore, generative AI in Healthcare company can accelerate MRI scan times, which is particularly beneficial for patients who cannot remain still for extended periods.
Personalized Medicine
AI is transforming healthcare through the personalization of medicine. By analyzing a patient’s genomic data, Artificial intelligence in healthcare can identify genetic variations that may lead to diseases and recommend personalized treatments tailored to the patient’s unique genetic profile. This approach, known as precision medicine, has the potential to revolutionize healthcare by enabling more individualized and targeted treatments.
For example, AI in Healthcare companies can analyze a patient’s genetic data to pinpoint specific mutations causing cancer. This detailed analysis can then be used to suggest personalized treatment options, such as drugs specifically designed to target those genetic mutations.
Diagnosis and Treatment Applications
For the past 50 years, the diagnosis and treatment of diseases have been central to the application of artificial intelligence (AI) in healthcare. Early rule-based systems showed potential for accurate diagnosis and treatment but were not widely adopted in clinical practice. These systems were not significantly more accurate than human diagnosticians and were challenging to integrate with clinician workflows and health record systems.
Whether rule-based or algorithmic, implementing AI in healthcare company for diagnosis and treatment plans often faces difficulties in harmonizing with clinical workflows and electronic health record (EHR) systems. Integration issues within healthcare organizations have posed a more significant barrier to the widespread adoption of AI than the accuracy of the AI’s suggestions. Many AI-powered diagnostic and treatment tools from medical software vendors are standalone solutions that address specific areas of care. Some EHR software vendors have started incorporating limited healthcare analytics functions with AI into their products, but these efforts are still in the early stages.
To fully leverage AI in healthcare using standalone EHR systems, providers must either undertake substantial integration projects themselves or utilize third-party vendors with AI capabilities that can integrate with their EHR systems.
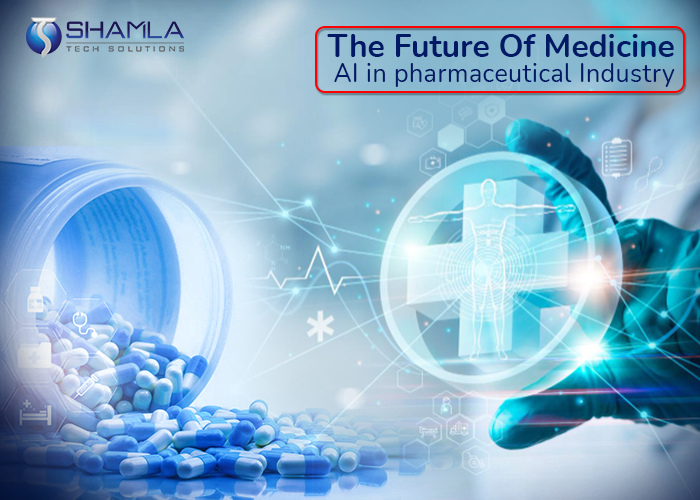
Administrative Applications
Artificial intelligence (AI) is revolutionizing many administrative aspects of medical care. By automating routine tasks such as data entry, claims processing, and appointment scheduling, AI can free up valuable time for healthcare providers and organizations, allowing them to focus more on patient care and revenue cycle management. Additionally, AI has the potential to reduce human error by providing faster, more accurate reviews of health records, medical imaging, claims processing, and test results.
AI in healthcare empowers medical professionals with greater control over their workflow, enabling them to deliver higher-quality patient care while maintaining budget efficiency. By analyzing patients’ medical histories and delivering better and faster results, AI is reshaping the way healthcare providers deliver care, allowing them to devote more time and resources to their patients. As AI leads the charge in improving patient care, medical professionals can focus on providing quality care, confident in the time and cost savings afforded by AI-powered administrative tasks.
Ultimately, AI in healthcare company offers a streamlined approach for healthcare providers to deliver better and faster patient care. By automating mundane administrative tasks, AI helps medical professionals save time and money while giving them more control over their workflow.
AI in Pharma
Drug Design & Optimization
In the pharmaceutical industry, AI is revolutionizing drug design and optimization. By analyzing chemical structures, AI in Pharmaceutical industry generates new compounds with desired properties, such as increased potency or reduced toxicity. This accelerates the drug discovery process, potentially leading to the development of more effective and safer drugs.
Clinical Trial Design
AI also plays a crucial role in clinical trial design. By generating synthetic patient data, AI can simulate clinical trials and provide insights into potential outcomes. This enables pharmaceutical companies to make more informed decisions about which drugs to bring to market and how to design more efficient and effective clinical trials.
Drug Repurposing
Generative AI can analyze existing drugs and their targets to suggest new therapeutic uses. This can save pharmaceutical companies time and money in the drug development process and potentially lead to new treatments for previously untreatable diseases. For example, Insilico Medicine, an AI-driven drug discovery company, received an FDA orphan drug designation for a drug candidate designed to treat idiopathic pulmonary fibrosis (IPF). Using AI-powered generative chemistry, the company simulated the structure of small molecules to identify a new compound with the potential to treat IPF.
AI and Market Access
AI & Pharma Marketing
AI has the potential to revolutionize pharmaceutical marketing by analyzing large amounts of data on healthcare provider (HCP) behaviour. Generative AI in pharmaceutical industry helps companies develop more targeted and effective marketing strategies, identifying the most effective channels, tailoring messages to specific segments, and predicting the success of marketing campaigns.
AI can identify rare disease patients and predict whether a doctor is likely to change their prescribing habits. It can also provide personalized recommendations to doctors and patients based on real-time data analysis. For instance, Novo Nordisk has incorporated AI into its pens, which track, monitor, and provide health predictions and advice, offering a comprehensive view of a patient’s health.
Generative AI is also a powerful tool for pharmaceutical marketers, enabling the rapid development of content such as articles, social media posts, graphics, and video tutorials. However, it is essential to use AI cautiously, as it can perpetuate biases, target vulnerable populations, or produce nonsensical information. Human oversight is critical to ensure ethical practices and accurate data use. With appropriate measures, generative AI can significantly benefit pharmaceutical marketing, resulting in cost savings, faster production, and improved idea generation.
Benefits of AI in Healthcare:
AI in Diagnostics
Examples of AI in healthcare are diverse and impactful. Beyond IBM’s Watson Health, Google’s DeepMind Health project demonstrated the ability to diagnose eye diseases from retinal scans with accuracy comparable to human experts. These pioneering projects showcased AI’s potential to revolutionize diagnostics and personalized medicine.
Patient Care Management
AI applications extend beyond diagnostics to reshape patient care management, drug discovery, and healthcare administration. In patient care, AI-driven chatbots and virtual health assistants provide 24/7 support and monitoring, enhancing patient engagement and adherence to treatment plans.
Predictive Analytics
Another significant impact of AI in healthcare is in predictive analytics. AI systems can analyze patterns in a patient’s medical history and current health data to predict potential health risks. This predictive capability enables healthcare providers to offer proactive, preventative care, leading to better patient outcomes and reduced healthcare costs.
Administrative Efficiency
AI streamlines various processes within healthcare facilities. From scheduling appointments to processing insurance claims, AI automation reduces administrative burdens, allowing healthcare providers to focus more on patient care. This improves operational efficiency and enhances the overall patient experience.
AI Technological Revolution
The rise of AI in healthcare has been a gradual but steady journey, catalyzed by technological advancements and the increasing demand for improved healthcare delivery. The integration of AI in medical field has brought about a paradigm shift, making healthcare more efficient, accurate, and personalized. As AI technology continues to evolve, its role in healthcare is set to become even more significant, further solidifying its status as an indispensable tool in modern medicine. This journey from a novel concept to a fundamental aspect of healthcare exemplifies a technological revolution, promising better health outcomes for all.
Benefits of Deploying AI in Pharma:
The benefits of using generative AI in the pharmaceutical industry extend far beyond the areas mentioned above, impacting the entire process from manufacturing to marketing.
Streamlined Manufacturing:
- AI optimizes production processes by identifying the most efficient and cost-effective manufacturing methods, thereby reducing waste and increasing yield.
Improved Patient Outcomes:
- AI can analyze patient data to identify patterns and correlations, enabling physicians to make more accurate diagnoses and develop more effective treatment plans.
Enhanced Regulatory Compliance:
- AI ensures compliance with regulatory requirements by analyzing data and identifying potential issues before they become problematic, thus reducing the risk of regulatory non-compliance.
Improved Supply Chain Management:
- Demand Prediction: AI can analyze data on historical sales, weather patterns, population demographics, and other factors to predict future drug demand. This allows pharmaceutical companies to plan production and distribution more accurately, avoiding overstocking or understocking.
- Real-Time Monitoring: AI monitors supply chain operations in real-time, identifying potential bottlenecks and disruptions. This enables pharmaceutical companies to proactively address issues, preventing delays in drug delivery.
- Inventory Optimization: AI analyzes data on sales patterns, expiration dates, and other factors to optimize inventory levels. This helps reduce waste by preventing drugs from expiring on the shelf while ensuring sufficient inventory to meet demand.
- End-to-End Visibility: AI provides end-to-end visibility across the supply chain, from raw materials to finished products. This helps identify gaps or inefficiencies and enables pharmaceutical companies to take corrective action before problems arise.
Immediate and Personalized Sales and Marketing:
- AI can personalize the customer experience, significantly enhancing customer engagement and strengthening the pharma-HCP (healthcare provider) relationship. This leads to a more effective and personalized approach to sales and marketing efforts.
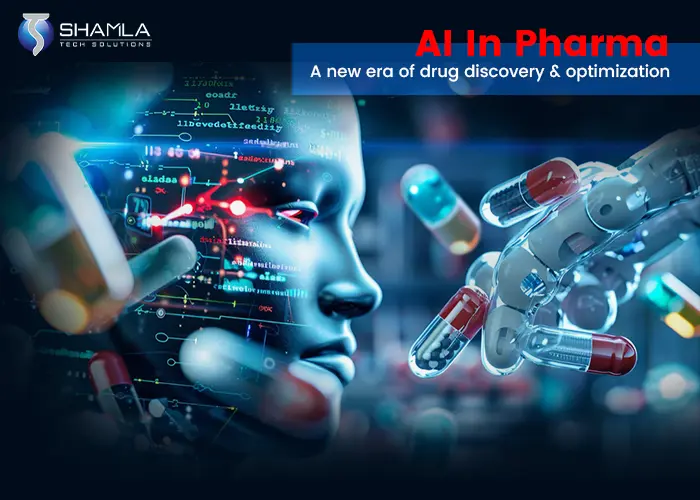
The Future of Artificial Intelligence in Healthcare:
During the 2024 Precision Med TriConference, Mara Aspinall of Illumina Ventures engaged in a fireside chat with Eric Topol, an esteemed executive VP, and professor at Scripps Translational Science Institute, to discuss the convergence of precision medicine and artificial intelligence (AI). Topol, a prolific author with three books and over 1,200 peer-reviewed publications, is a leading figure in digital medicine. He strongly advocates for AI in medicine and healthcare, though he questions the readiness of the medical community for this significant shift.
Topol remarked, “I believe this will be the most significant transformation in medical history, yet we are only at the beginning. We lack the necessary solid evidence from real-world applications. Until we obtain this, we must approach our advances with caution. Nevertheless, the indicators of progress are emerging rapidly.”
With the advent of advanced AI technologies in medical tools and intelligent algorithms capable of interpreting large datasets, the potential for AI in healthcare is limitless. Deep learning AI can expedite disease detection, provide personalized treatment plans, and automate processes such as drug discovery and diagnostics. It also holds promise for improving patient outcomes, enhancing safety, and reducing healthcare delivery costs.
The future of AI in healthcare is undoubtedly bright and brimming with possibilities for further innovation. As we progress into a more interconnected digital era, AI in healthcare industry is the valuable asset, potentially reshaping how doctors treat patients and deliver care. With such immense potential, it is clear that AI in healthcare promises advancements, improved health outcomes, and better patient experiences.
The primary obstacle for AI in healthcare is not its efficacy, but rather its integration into everyday clinical practice. Over time, medical professionals may gravitate toward roles that require distinctly human skills, particularly those involving advanced cognitive functions. The only healthcare providers who might not fully benefit from AI advancements are those who choose not to embrace its use.
Challenges in Deploying AI in Healthcare and Pharma:
The integration of artificial intelligence (AI) into healthcare and pharmaceuticals holds immense potential. From accelerating drug discovery to personalizing patient treatment plans, AI can revolutionize how healthcare is delivered. However, the deployment of AI in these fields comes with a host of challenges that must be addressed to fully realize its benefits. This blog will explore the key challenges in deploying AI in healthcare and pharma, including data quality and availability, regulatory compliance, integration with existing systems, ethical considerations, and the need for a skilled workforce.
Data Quality and Availability
AI systems thrive on data. In healthcare and pharma, the quality and availability of data are paramount for AI to function effectively. However, data in these fields are often fragmented, unstructured, and stored in various formats across different systems. This fragmentation makes it challenging to gather comprehensive datasets necessary for training AI models.
Moreover, healthcare data includes sensitive patient information, which must be handled with the utmost care to maintain privacy and comply with regulations such as the Health Insurance Portability and Accountability Act (HIPAA) in the United States and the General Data Protection Regulation (GDPR) in Europe. Ensuring data security while making it accessible for AI training and deployment is a significant challenge.
Regulatory Compliance
The healthcare and pharmaceutical industries are heavily regulated to ensure patient safety and efficacy of treatments. Introducing AI into these sectors requires navigating complex regulatory landscapes. Regulatory bodies such as the Food and Drug Administration (FDA) in the United States and the European Medicines Agency (EMA) have stringent requirements for the validation and approval of new technologies.
AI systems must demonstrate not only their effectiveness but also their reliability and safety. This involves rigorous testing, clinical trials, and continuous monitoring post-deployment. The regulatory approval process can be time-consuming and expensive, posing a significant hurdle for the rapid adoption of AI technologies.
Integration with Existing Systems
Healthcare facilities and pharmaceutical companies often operate with legacy systems that were not designed to integrate with modern AI technologies. These systems may include electronic health records (EHRs), laboratory information management systems (LIMS), and various other software used for clinical and administrative purposes.
Integrating AI into these existing systems requires significant technical expertise and investment. It involves developing interfaces, ensuring interoperability, and sometimes overhauling entire IT infrastructures. The complexity and cost of these integration efforts can be a major barrier to AI deployment.
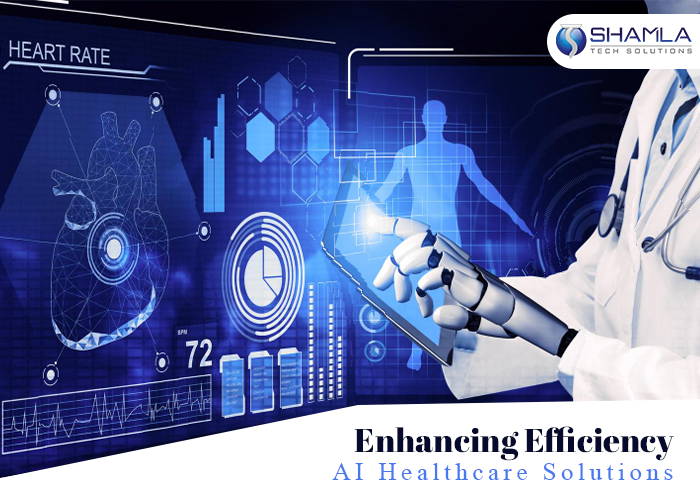
Ethical Considerations
AI in healthcare and pharma raises numerous ethical questions. These include concerns about data privacy, algorithmic bias, and the potential for AI to exacerbate existing health disparities. For instance, AI models trained on data from specific populations may not perform well on data from underrepresented groups, leading to biased or inaccurate results.
Ensuring ethical AI deployment requires establishing guidelines and frameworks for responsible AI use. This includes implementing transparency measures, ensuring diversity in training datasets, and continuously monitoring AI systems for biases and unintended consequences. Balancing the benefits of AI with ethical considerations is a delicate and ongoing challenge.
Need for a Skilled Workforce
Deploying AI in healthcare and pharma requires a workforce skilled in both domains. This includes data scientists who understand the complexities of healthcare data, as well as AI healthcare company professionals who can interpret AI-generated insights and make informed decisions.
There is a growing demand for professionals with interdisciplinary expertise in AI and healthcare. Educational institutions and industry stakeholders must collaborate to develop training programs and curricula that equip the workforce with the necessary skills. Bridging the knowledge gap and fostering collaboration between technologists and AI healthcare providers is crucial for successful AI deployment.
Addressing Technological Limitations
While AI has shown great promise, it is not without its technological limitations. AI models can be complex and opaque, making it difficult to understand their decision-making processes. This “black box” nature of AI can hinder trust and acceptance among healthcare AI companies professionals and regulators.
Efforts are being made to develop explainable AI (XAI) systems that provide insights into how AI models arrive at their conclusions. However, achieving a balance between model complexity and interpretability remains a challenge. Additionally, AI systems require continuous updates and improvements to adapt to new medical knowledge and data, necessitating ongoing investment in research and development.
Cost and Financial Barriers
Implementing AI solutions in healthcare and pharma can be expensive. The costs associated with acquiring technology, integrating it with existing systems, training staff, and maintaining AI infrastructure can be prohibitive, especially for smaller organizations and facilities with limited budgets.
Securing funding for AI projects often requires demonstrating a clear return on investment (ROI), which can be challenging given the uncertainties and long timelines associated with AI implementation. Financial barriers can slow down the adoption of AI, particularly in resource-constrained settings.
Resistance to Change
AI Healthcare and pharmaceutical industries have long-established practices and protocols. Introducing AI into these environments requires a cultural shift, which can be met with resistance from stakeholders accustomed to traditional methods.
Overcoming resistance to change involves educating AI healthcare professionals about the benefits and limitations of AI, addressing their concerns, and involving them in the development and deployment process. Building trust and fostering a culture of innovation are essential for gaining acceptance and support for AI initiatives.
Ensuring Interoperability
For AI to be effective in healthcare and pharma, it must seamlessly interact with a variety of systems and data sources. Ensuring interoperability is a complex challenge that requires standardizing data formats, protocols, and communication interfaces across different platforms and technologies.
Efforts to promote interoperability include the development of international standards and frameworks, such as Fast AI Healthcare Interoperability Resources (FHIR) and Digital Imaging and Communications in Medicine (DICOM). Achieving widespread interoperability is a critical step toward realizing the full potential of AI in healthcare and pharma.
The Impact of AI on the Drug Supply Chain
Here’s how AI is making a significant difference:
End-to-End Process Visibility
AI provides comprehensive visibility across the entire supply chain, enabling stakeholders to:
- Spot Process Inefficiencies: Identify bottlenecks and areas for improvement to streamline operations.
- Generate Real-Time Actionable Insights: Utilize data to make informed decisions quickly, enhancing responsiveness and agility.
- Improve Efficiency and Control: Ensure smooth and controlled operations through enhanced transparency.
- Optimizing Pharma Inventory Management
AI helps maintain optimal inventory levels, improving decision-making processes by:
- Keeping Inventory Low: Reduce excess stock while ensuring sufficient supply to meet demand.
- Enhancing Warehousing and Demand Decisions: Analyze data to predict inventory needs accurately.
- Preventing Stock-Ups and Shortages: Plan effectively to avoid overstocking or running out of essential drugs.
- Optimizing for Predictive Maintenance
AI-driven predictive maintenance maximizes supply chain efficiency by:
- Producing Actionable Predictions: Anticipate equipment failures and maintenance needs before they become critical.
- Orchestrating Maintenance Efforts: Coordinate maintenance schedules to minimize downtime and disruptions.
- Enhancing Overall Efficiency: Maintain a smooth, efficient supply chain by addressing issues proactively.
- Medicine Demand Forecasting, Logistics, and Stock Management
AI significantly improves forecasting and logistics management by:
- Demand Forecasting and Proactive Manufacturing: Predict demand trends and adjust production schedules accordingly.
- Managing Transportation Capacity: Plan and allocate transportation resources effectively to meet delivery timelines.
- Advanced Transportation Management: Utilize AI for efficient management of cold chain logistics, ensuring the integrity of temperature-sensitive drugs.
- Smart Automation
AI-powered automation increases accuracy and efficiency in supply chain operations by:
- Generating Strategic Insights from IoT Sensors: Leverage data from sensors to gain valuable operational insights.
- Automated Quality Assurance: Use sensors and cameras to ensure consistent product quality and compliance.
- Improving Operations: Enhance accuracy, repeatability, and throughput while reducing human errors and maintaining high-quality standards.
- A Secure Process Resistant to Counterfeit
AI ensures the security and integrity of pharmaceutical products through:
- Quality Assurance for Every Batch: Implement automated quality checks to maintain product standards.
- Automated Compliance Systems: Ensure adherence to regulatory requirements with minimal manual intervention.
- Shielding Against Counterfeits: Create a secure system that protects against counterfeit and substandard drugs, ensuring patient safety.
By integrating AI into the drug supply chain, pharmaceutical companies can achieve greater efficiency, accuracy, and security. These advancements lead to better resource management, improved patient outcomes, and a more resilient supply chain.
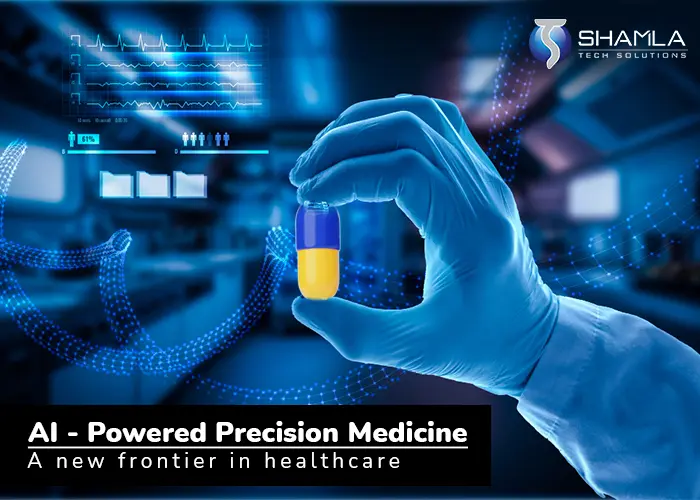
Conclusion
The transformative potential of artificial intelligence healthcare companies and artificial intelligence in pharmacy is undeniable. From streamlining drug discovery and enhancing medical imaging to personalizing patient care and optimizing supply chain management, AI is revolutionizing these industries. However, the deployment of AI is not without its challenges. Issues such as data quality, regulatory compliance, integration with legacy systems, ethical considerations, and the need for a skilled workforce must be addressed to fully harness AI’s capabilities.
Despite these hurdles, the benefits of AI are profound. In healthcare, AI offers improved diagnostic accuracy, personalized treatment plans, and enhanced patient care management. It also reduces administrative burdens, allowing healthcare providers to focus more on patient care. In the pharmaceutical industry, AI accelerates drug design, optimizes clinical trial designs, repurposes existing drugs, and ensures regulatory compliance. Additionally, AI enhances supply chain efficiency through improved visibility, predictive maintenance, and smart automation, safeguarding the integrity of pharmaceutical products.
As we move forward, it is crucial for stakeholders in healthcare and pharma to collaborate, innovate, and adapt to the evolving AI landscape. By addressing the challenges and embracing the opportunities AI presents, these industries can achieve significant advancements, leading to better health outcomes, increased efficiency, and improved patient experiences. The journey of AI from a novel concept to an integral component of healthcare and pharma exemplifies a technological revolution, promising a future filled with innovation, enhanced patient care, and optimized pharmaceutical processes.